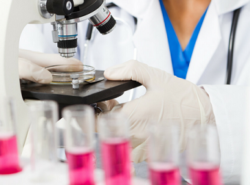
Effective Artificial Intelligence in Breast Cancer Screening
Published: 05/8/22 7:03 AM
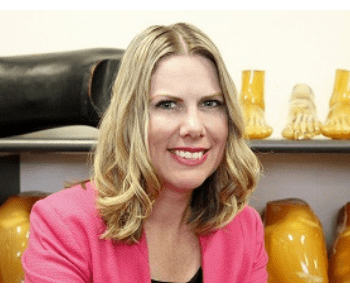
Sarah Lewis
Project Description: During routine breast screening by BreastScreen Australia, each woman’s mammogram is assessed by at least two qualified radiologists (doctors specialising in medical imaging) in an approach known as double-reading. However, this approach can place significant strain on human resources due to a current shortage of breast imaging radiologists in Australia. This study will investigate the effectiveness and accuracy of artificial intelligence (AI) tools in breast screening to work alongside radiologists and harness the power of big data sets and AI.
Why This Work is Needed: Whilst mammographic screening programs are a highly effective method to detect early breast cancer, they require physical high resources and expert knowledge to interpret the images. Recently, some stand-alone AI systems have reported high rates of accuracy, comparable to the level of experienced radiologists. This study will investigate how AI tools can be optimally implemented to improve detection and reduce workload in this process, working in partnership with radiologists as a pre-screener, triage or supportive tool.
Expected Outcomes: This study will provide important critical knowledge on the optimal pathways to integrate AI tools in double-reading or new reading strategies to produce the best screening outcomes for Australian women. Successful implementation of this project will place Australia as a worldwide leader of AI in this area, with Australian women directly benefitting from the best deployment of AI in breast cancer screening.
Project Details
BreastScreen Australia (BSA), the population-based mammography screening program for early detection of breast cancer, currently screens more than 1.8 million women in each two-year cycle. For each woman screened at BSA, two qualified radiologists will independently review the images, with consensus via a third arbitrator radiologist. This double-reading strategy puts significant strain on existing human resources and can lead to higher false positive results, impacting upon consumer anxiety. Some international screening programs employ single reading but with the trade-off of reduced sensitivity (ability to correctly identify patients with breast cancer).
A few recent AI tools have achieved levels of accuracy comparable to experienced human radiologists in detecting breast cancer. This has led to a growing interest to use AI in screening practices, including as a potential replacement for the second reader or as an ‘early screener’, to help solve problems associated with radiologist workforce shortages and capitalise on the large data sets that screening mammography creates.
However, there are still questions to be answered before AI can be fully integrated into breast screening practices. For example, it is not known how radiologists and AI can interact and operate in a complementary way to produce the best screening outcomes for early detection of breast cancer or if AI might have a de-skilling effect. Therefore, it is essential to determine how to optimally integrate AI in routine screening practices in partnership with the radiology community.
To address this knowledge gap, Professor Sarah Lewis from the University of Sydney, will lead a study to investigate whether AI could be best used as a second reader to radiologists, as a tool for pre-screening before a radiologist’s viewing, or as a tool to help prioritise suspicious cases. Overall, this study will help answer hard questions about how AI can be best deployed in breast screening programs towards achieving optimal screening outcomes for Australian women.